Home Improvement
Trenton 126lb Mouse Hole Anvil Identification: History, Innovation & Modern Impact
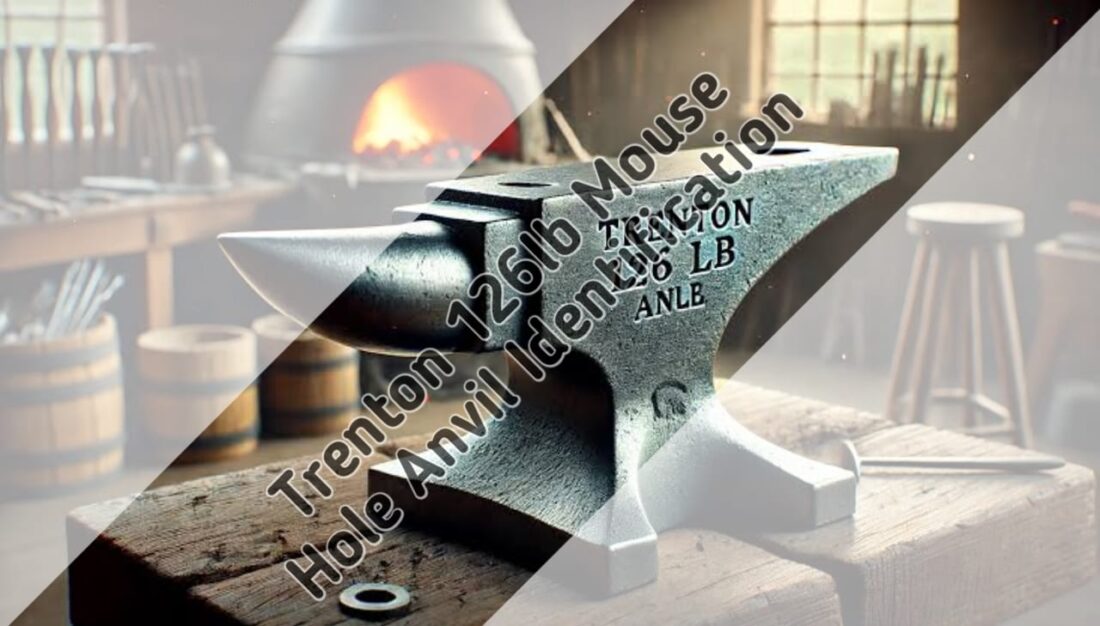
Introduction to Trenton 126lb Mouse Hole Anvil Identification
The term “trenton 126lb mouse hole anvil identification” goes beyond a mere technical description—it tells the story of a unique industrial artifact that has captivated the interest of historians, metalworkers, and collectors for generations. Weighing 126 pounds, this anvil symbolizes a period when robust construction and innovative design converged to produce tools that were both practical and artful.
Its intriguing “mouse hole” detail hints at specialized design choices that set it apart from ordinary anvils, prompting further exploration into its origin, structure, and manufacturing methods. In an era that increasingly values the preservation of industrial heritage, understanding every aspect of this iconic tool is essential.
This article embarks on a comprehensive journey, covering its historical roots, engineering ingenuity, material composition, authentication processes, contemporary relevance, collector perspectives, and prospects for future integration with modern technology. Each section is organized into clear, focused paragraphs to offer readers a complete and engaging insight into the enduring legacy of the trenton 126lb mouse hole anvil identification.
Historical Context of Trenton 126lb Mouse Hole Anvil Identification
The history behind the trenton 126lb mouse hole anvil identification is both layered and fascinating. Emerging during the height of American industrial innovation, this anvil represents a time when manufacturing excellence and detailed craftsmanship were at the forefront of production. Early manufacturers in Trenton were renowned for blending robust construction methods with creative design ideas, giving birth to an artifact that would eventually become a symbol of technical prowess and industrial strength.
Historical records suggest that the evolution of this anvil was marked by continuous experimentation and improvement. Small local workshops and larger foundries played a role in refining its design, ensuring that each unit not only served a functional purpose but also embodied the spirit of craftsmanship and quality. Over time, the distinct “mouse hole” feature evolved into a unique hallmark that distinguished these anvils from more conventional models.
This detail likely served both as a functional element during forging processes and as an unmistakable indicator of authenticity. By reflecting the socioeconomic conditions and technological advances of its era, the trenton 126lb mouse hole anvil identification has earned its place as a celebrated piece of industrial history.
Design and Engineering of Trenton 126lb Mouse Hole Anvil Identification
At the core of the trenton 126lb mouse hole anvil identification lies a masterful fusion of design and engineering. Artisans of the time meticulously engineered each anvil to ensure that its form was as robust as its function. The layout of the anvil demonstrates an advanced understanding of weight distribution, durability, and ergonomic efficiency.
A standout feature is its “mouse hole” detail—a design innovation that was far more than decorative. This distinctive element was likely conceived to improve the tool’s utility during metalworking, facilitating a smoother workflow while maintaining structural integrity. Multiple iterations and rigorous testing allowed engineers to perfect the balance between aesthetic appeal and functional performance.
Every curve, edge, and contour was crafted with precision, reflecting both an artistic vision and a deep practical understanding of material properties. Such design considerations have not only ensured the anvil’s longevity under harsh industrial conditions but have also influenced modern metalworking tools. Today, the enduring legacy of this engineering marvel continues to inspire contemporary designers and engineers who seek to combine form with functionality.
Material Composition and Craftsmanship of Trenton 126lb Mouse Hole Anvil Identification
The exceptional durability of the trenton 126lb mouse hole anvil identification is rooted in its superior material composition and skilled craftsmanship. Forged primarily from high-grade cast iron, this anvil was produced using a careful selection of alloys that provided an optimal balance between toughness and heat retention. This thoughtful choice of materials ensured that the anvil could withstand repetitive, high-impact use without warping or degrading.
Beyond its robust construction, the craftsmanship behind each anvil is noteworthy. Traditional forging techniques were paired with innovative casting processes to produce a tool that is as aesthetically pleasing as it is functional. The integration of the “mouse hole” into its design is a clear demonstration of the artisan’s attention to detail—each anvil not only met rigorous quality standards but also carried an unmistakable mark of excellence.
From the initial mixing of alloys to the final tempering and finishing touches, every step was executed with precision. These meticulous processes allowed for the production of an anvil that has withstood the test of time, preserving both its physical integrity and its historical significance.
Authentication and Verification of Trenton 126lb Mouse Hole Anvil Identification
Ensuring the authenticity of the trenton 126lb mouse hole anvil identification is critical for historians, collectors, and industrial enthusiasts alike. Experts employ a multifaceted approach to verify each anvil’s origin and integrity, combining traditional examination methods with modern scientific techniques.
Initial assessments typically involve thorough visual inspections where experts scrutinize every design detail, surface marking, and subtle nuance that might indicate alterations or reproductions. These observations are then cross-referenced with archival manufacturing records and historical documentation.
To complement this, advanced methods such as X-ray fluorescence and metallographic analysis are used to assess the metal composition, ensuring it aligns with the original specifications. Comparative analysis with other authenticated pieces further strengthens the verification process. This rigorous authentication not only protects the historical value of these anvils but also provides assurance to collectors and museums about the genuineness of each artifact. Through a combination of detailed scrutiny and scientific precision, the legacy of the trenton 126lb mouse hole anvil identification is carefully preserved for future generations.
Contemporary Relevance and Applications of Trenton 126lb Mouse Hole Anvil Identification
Despite its roots in historical manufacturing, the trenton 126lb mouse hole anvil identification continues to have practical and symbolic importance in today’s industrial landscape. Modern artisans and blacksmiths have rediscovered the value of this time-honored tool, incorporating it into both functional metalworking practices and as a source of inspiration.
Its durable construction and well-balanced design offer practical benefits that enhance traditional forging techniques, while its historical significance elevates its status as a collector’s item. Museums and private collections around the world showcase these anvils as embodiments of industrial ingenuity and cultural heritage.
Moreover, ongoing research into digital archiving and 3D modeling has allowed for detailed documentation of the anvil’s design, making its study accessible to a broader audience. In professional circles, the design principles evident in the trenton 126lb mouse hole anvil identification continue to influence modern tool development, bridging the gap between past innovations and current industrial trends.
Collector Perspectives and Preservation Efforts for Trenton 126lb Mouse Hole Anvil Identification
Collectors and preservationists have long recognized the trenton 126lb mouse hole anvil identification as a valuable piece of industrial history. For these enthusiasts, the anvil represents not only an essential tool from a bygone era but also a work of art that embodies the ingenuity and dedication of its makers.
Extensive research into the anvil’s provenance, design evolution, and manufacturing techniques is common among collectors, who invest considerable effort in verifying its authenticity and historical significance. Collaborative projects involving historians, industry experts, and restoration specialists are frequently undertaken to document and preserve these artifacts.
Through careful restoration and conservation, the physical and historical integrity of the anvil is maintained, ensuring it remains a testament to early industrial excellence. Public exhibitions, museum displays, and digital archives have all played a role in sharing the story of the trenton 126lb mouse hole anvil identification with a wider audience, thus preserving its legacy for future generations.
Future Perspectives and Technological Integration in Trenton 126lb Mouse Hole Anvil Identification
Looking forward, the future of the trenton 126lb mouse hole anvil identification is being shaped by a blend of historical reverence and modern technological advances. Researchers and industry experts are increasingly leveraging digital tools to capture detailed records of these artifacts. Technologies such as 3D scanning, augmented reality, and advanced imaging techniques are now being used to create comprehensive digital models that allow for remote study and analysis.
These innovations not only facilitate meticulous preservation and restoration efforts but also enhance our understanding of the anvil’s intricate design and engineering. As contemporary design and manufacturing continue to draw inspiration from historical methods, the principles exemplified by this anvil remain relevant in modern industrial practices.
The ongoing dialogue between traditional craftsmanship and technological progress promises to open new avenues for both academic research and practical applications, ensuring that the legacy of the trenton 126lb mouse hole anvil identification continues to inform and inspire future generations.
Conclusion
The trenton 126lb mouse hole anvil identification stands as a powerful symbol of industrial innovation and enduring craftsmanship. Its rich history, thoughtful design, and meticulous construction have made it a revered artifact in both historical and modern contexts. Through rigorous authentication and preservation efforts, experts ensure that each anvil retains its unique identity and significance.
Today, its influence is seen not only in traditional metalworking practices but also in modern industrial design, where its legacy continues to inspire innovation. As we look to the future, the integration of advanced technologies promises to further illuminate the fascinating story behind this iconic tool, preserving its heritage while paving the way for new discoveries in the realm of industrial history.
Also Read:
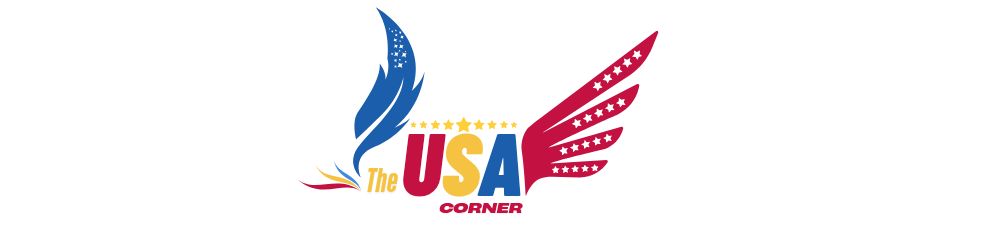
-
Uncategorized6 days ago
Spring Ferraz A-102914 Righ: A Comprehensive Guide to Specs, Design, Performance, Installation, Applications & Future Trends
-
Blog6 days ago
Anaëlle Benoist d’Anthenay: Early Life, Education, Art & Cultural Impact
-
Lifestyle5 days ago
Lillienu: Bridging History, Innovation, Technology, Market Trends, and Sustainability for a Future-Ready Industry
-
Tech3 days ago
Ultimate Guide to mihomo-linux-amd64-v1.18.5.gz: Installation, Features, Performance Optimization,, Security & Troubleshooting